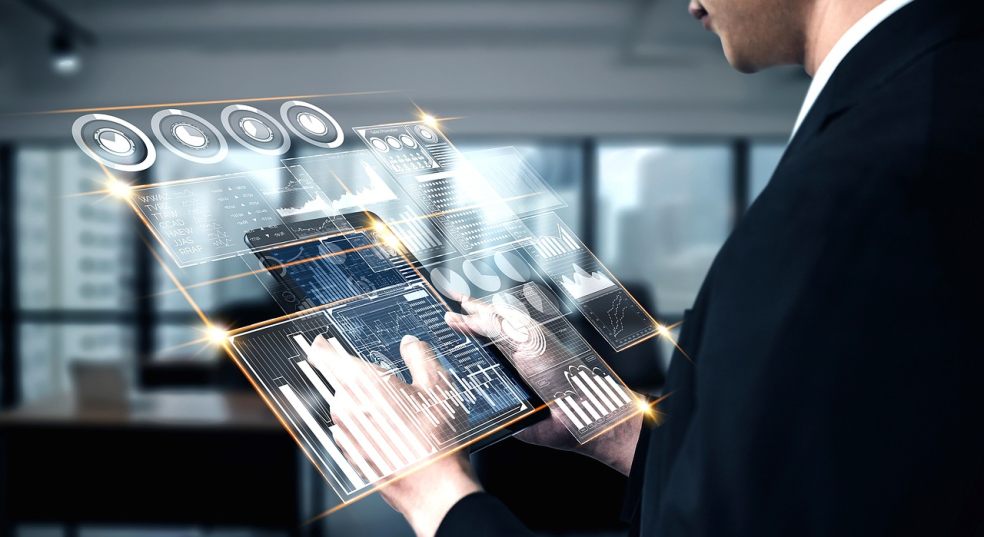
The Role of Math AI in Strategic Pricing and Revenue Management
In the competitive landscapes of today, pricing strategies and revenue management are no longer reliant solely on intuition and spreadsheets. Math takes center stage as AI surprisingly transforms into a powerhouse tool. Businesses looking to give their pricing a serious boost can turn to Math AI, which expertly melds data analysis with algorithmic might to drive revenue growth. What drive's this magic, and how does it all fit together? So what exactly makes it tick?
Decoding Math AI in Pricing and Revenue Management
Math AI zeroes in on the data, mining it for patterns, predicting what's next, and optimizing choices with surgical precision. AI stands out from traditional approaches by digging through vast amounts of data to reveal surprising insights. Without the constraints of human interpretation, AI is free to uncover connections that might have otherwise remained hidden. For instance, a 2023 survey by Gartner revealed that companies using AI for pricing saw a 12% increase in revenue on average, compared to their non-AI-using counterparts.
The thing that separates Math AI from the pack is its fluid intelligence - it can assimilate new knowledge and tweak its strategy on the fly. The more data machine learning algorithms crunch, the more refined their pricing strategies become, capturing the subtleties of a dynamic market. With pricing, one wrong move can trigger a profits freefall; agility is what saves the day, allowing you to correct course and stay ahead.
Strategic Pricing: The AI Advantage
Strategic pricing, a critical component of revenue management, involves setting prices based on perceived value, market demand, and competitor behavior. Finding the perfect balance in pricing can require solving complex problems. However, an AI-powered math solver makes it easy. The app can solve math problems in seconds using just a photo. Math AI enhances this process in several ways:
- Dynamic Pricing Adjustments: AI systems can calculate optimal price points in real time. For example, e-commerce giants like Amazon utilize AI-driven algorithms to adjust prices 2.5 million times daily, ensuring that their products remain competitively priced without sacrificing profit margins.
- Predictive Analytics: By analyzing historical sales data and external factors such as seasonality or economic trends, Math AI predicts how customers will respond to price changes. For example, a hotel chain might leverage AI to anticipate demand during a holiday season and increase room rates accordingly, capturing additional revenue.
- Segmentation at Scale: Customer segmentation is vital for personalized pricing. AI enables businesses to group customers into micro-segments, identifying unique willingness-to-pay thresholds. Airlines, for instance, use such AI systems to adjust ticket prices based on factors like booking time and travel distance.
Revenue Management: Data-Driven Decision Making
Revenue management extends beyond pricing—it involves optimizing the entire sales funnel. Math AI empowers businesses with tools that extend across inventory management, demand forecasting, and profit maximization.
- Inventory Optimization: For industries with perishable inventory (e.g., hotels, airlines), managing supply is crucial. AI models predict occupancy rates or seat bookings with remarkable precision. A study by McKinsey showed that AI-driven revenue management systems improved inventory utilization by 20-30%, reducing waste and boosting profitability.
- Demand Shaping: AI systems help companies influence customer behavior. By simulating different pricing scenarios, businesses can identify which promotional campaigns or bundling strategies will drive the most revenue. For example, a streaming service might use AI to determine the price elasticity of its subscription tiers, finding the sweet spot that maximizes subscriber growth and revenue simultaneously.
- Competitive Benchmarking: Math AI continuously monitors competitors’ pricing strategies. This information enables businesses to maintain competitive pricing without resorting to aggressive discounts that could undermine long-term profitability.
Challenges and Ethical Considerations
Despite its advantages, integrating Math AI into pricing and revenue management isn’t without challenges. Algorithms are only as good as the data they consume. Poor data quality or biases in training sets can lead to inaccurate predictions or discriminatory pricing strategies.
Additionally, there are ethical concerns. For example, dynamic pricing might inadvertently exploit customers during emergencies or peak demand periods, as seen in controversies over ride-sharing surge pricing. To address these issues, businesses must balance profit goals with fairness and transparency.
Case Studies: Success in Action
Several companies have demonstrated the transformative power of Math AI in pricing and revenue management:
- Retail: Zara, a leader in fast fashion, uses AI-driven pricing to manage its limited inventory. The system predicts which items will sell quickly at full price and which will require markdowns, minimizing unsold stock.
- Hospitality: Marriott International implemented AI for dynamic room pricing, resulting in an 8% revenue increase in its first year of adoption. The system dynamically adjusted rates based on demand, competitor pricing, and booking windows.
- Transport: The logistics company UPS utilizes Math AI to optimize shipping rates. By analyzing fuel costs, route efficiency, and delivery urgency, UPS maximizes its revenue while maintaining customer satisfaction.
Conclusion
The role of Math AI in strategic pricing and revenue management is nothing short of revolutionary. However, with great power comes great responsibility—companies must wield AI ethically, ensuring fairness while pursuing profitability.
In a world where every penny counts, Math AI is no longer a luxury but a necessity. Its ability to analyze, predict, and optimize is changing the game, one algorithm at a time. And as technology advances, the question is not whether to adopt Math AI, but how fast you can implement it to stay ahead.